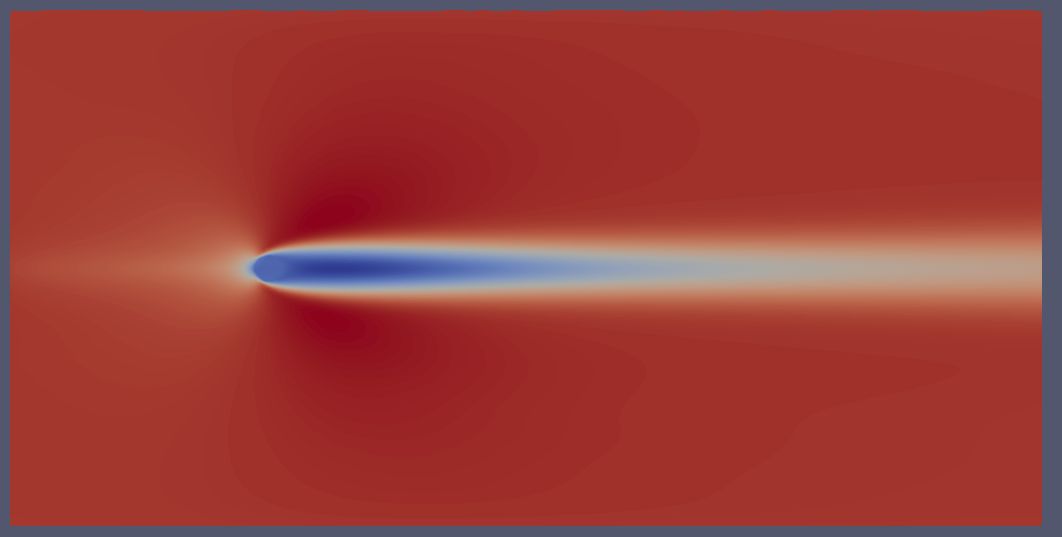
SUJET : 16 Juin 9h00 salle Coriolis de l'OMP : Séminaire par N. Loppi AI/HPC architect/NVIDIA : Physics-Informed Neural Networks (PINNs) with NVIDIA Modulus Sdk
Bonjour à tous,
ANITI, l'axe AOC/CIMI, l'atelier numérique de l'OMP, CALMIP, le CUTIS et le chantier ENVIA vous convie à un séminaire le 16 Juin à partir de 9h00 salle Coriolis de l'OMP.
Titre : Physics-Informed Neural Networks (PINNs) with NVIDIA Modulus: Application to external flow problems.
Intervenant : Niki Loppi, AI/HPC solutions architect, NVIDIA.
Programme :
09h00 - 09h30 : Accueil et pauses cafés.
09h30 - 10h30 : Présentation.
10h30 - 11h30 : Tour de table et Questions / Réponses.
Attention : Le séminaire sera uniquement en anglais et en présentiel.
Résumé : In this talk, we will review the fundamentals of Physics-informed Neural Networks (PINN) and explore the capabilities of NVIDIA Modulus framework for solving external flow problems that are common in various aero/hydrodynamic applications. Specifically, we will focus on how Modulus is able to train parameterised PINNs which, after training, can be used simulate different flow conditions almost instantaneously. Furthermore, we will also present how users can undertake unsteady (time-dependent) simulations using the moving time-window approach. Finally, we will show results for a parameterized unsteady case which demonstrates that PINNs are able to capture temporal dynamics across a range of Reynolds numbers.
Biographie : Niki is an AI/HPC solutions architect at NVIDIA, helping academic researchers to leverage NVIDIA’s technology stack through the NVIDIA AI Technology Center program. Prior to joining NVIDIA, he worked as a researcher in the Department of Aeronautics at Imperial College London, where he also obtained his PhD in Computational Fluid Dynamics. Specifically, his research focused on the development of high-order accurate numerical methods for solving large-scale incompressible fluid flow problems using massively parallel modern GPU architectures.